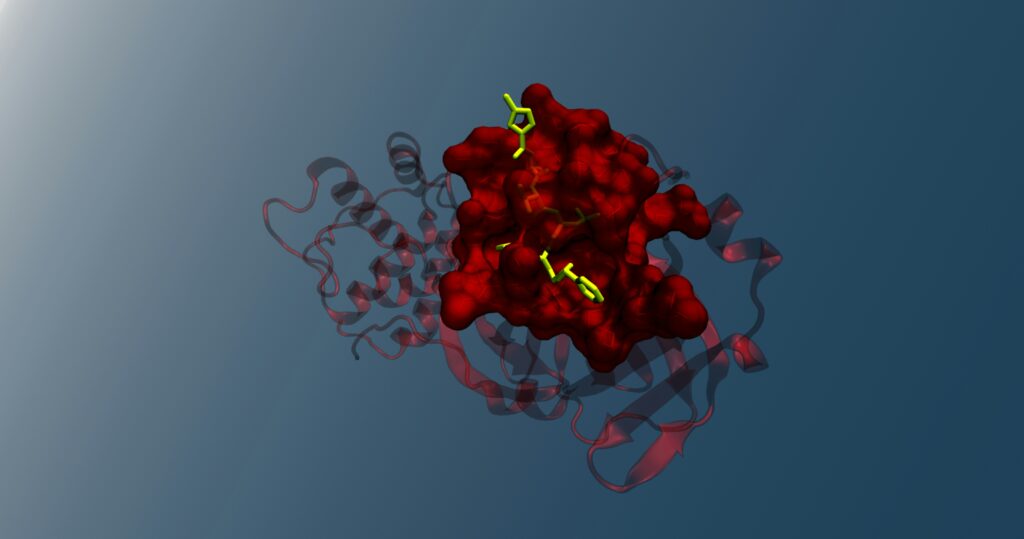
Vina GPU is an advanced molecular docking software that leverages GPU acceleration to increase the speed and efficiency of virtual screening workflows significantly. Built upon the popular AutoDock Vina framework, Vina GPU accelerates the docking process by running tasks on massively parallel GPU architectures, making it possible to screen millions (or even billions) of ligands against target proteins in a fraction of the time required by traditional CPU-based methods.
What is Vina GPU 2.1?
Vina GPU 2.1 offers three algorithmic approaches to molecular docking:
- AutoDock Vina GPU 2.1 – Provides balanced accuracy and performance.
- QuickVina W GPU 2.1 – Optimized for rapid docking.
- QuickVina2 GPU 2.1 – Focuses on high-throughput docking with reduced computational time.
Each method offers a different balance of speed and accuracy, allowing users to choose the best approach for their specific docking needs. Further information on the runtime and accuracy comparisons for each of the approaches for virtual screening can be found here.
Vina GPU 2.1 is particularly well-suited for high-throughput virtual screening (HTVS), structure-based drug discovery, and hit-to-lead optimization. Researchers can use it to screen large libraries of ligands quickly, identify promising candidate molecules, and evaluate ligand modifications to improve binding affinity.
Running Vina GPU 2.1 at scale presents several challenges. Deploying large-scale GPU clusters in the cloud can be prohibitively expensive, especially for billion-ligand-scale projects. Acquiring and maintaining a large number of GPUs is difficult due to availability and pricing fluctuations. Additionally, optimizing cloud infrastructure to balance cost and speed requires expertise and careful tuning, making it a complex task for most research teams.
Docking at a scale of billions of ligands introduces several challenges:
- Acquiring and distributing large-scale GPU resources can be difficult.
- Balancing cost and performance efficiently requires careful optimization.
- Managing complex cloud infrastructure at this scale demands advanced expertise.
Fovus addresses these challenges by intelligently optimizing HPC strategies and dynamically orchestrating cloud logistics using AI.
Introducing Fovus: Accelerating Vina GPU at Scale
Fovus is an AI-powered, serverless high-performance computing (HPC) platform designed to enhance performance, scalability, and cost efficiency for complex workloads like Vina GPU. By automating cloud logistics and optimizing HPC strategies, Fovus ensures sustained time-cost optimality amid evolving cloud infrastructures. The platform provides automated benchmarking to evaluate instance configurations, AI-driven optimization to ensure efficient computation, and multi-cloud auto-scaling to distribute workloads across regions dynamically. By leveraging spot instances with failover capabilities intelligently, Fovus further reduces costs while continuously adapting to evolving cloud infrastructure. Its serverless model eliminates all cloud management hassles, allowing single-command deployment with pay-per-use billing.
Key Benefits of Using Fovus
Fovus offers several advantages for running Vina GPU efficiently:
- Free Automated Benchmarking: Evaluates how different instance choices, system configurations (CPUs, GPUs, memory, and more), and parallel computing settings impact the runtime and cost of runs.
- AI-driven HPC Strategy Optimization: Automatically determines optimal instance choices, system configurations, and parallel computing settings, ensuring efficient and cost-effective computation.
- Dynamic Multi-Cloud-Region Auto-Scaling: Allocates optimal GPUs across multiple cloud regions and availability zones, efficiently scaling up GPU clusters for large-batch simulations.
- Intelligent Spot Instance Utilization: Intelligently utilizes spot GPUs based on availability and pricing dynamics, with spot-to-spot failover capabilities to minimize computation costs.
- Continuous Improvement: Automatically updates benchmarking data and refines HPC strategies as cloud infrastructure evolves.
- Serverless HPC Model: Eliminates all cloud management hassles through AI-driven automation, allowing for single-command or a few-click deployment, with users paying only for runtime.
Scaling Vina GPU with Fovus From Millions to Billions of Ligands
For smaller docking tasks with millions of ligands, CPU-based systems can still be practical. However, when scaling up to billions of ligands, the computational demands grow exponentially, making GPU acceleration essential. For efficient time-to-insight, CPU-based docking approaches typically run 100–1,000 dockings per CPU core, scaling out to 5000–10,000 CPU cores for handling the high-throughput virtual screening of millions of ligands. In contrast, with an AI-optimized HPC strategy, Vina GPU 2.1 on Fovus can dock 30,000 ligands on a single GPU as fast as 15 minutes, significantly reducing the overall runtime and cost. Equivalently, 1M or 1B ligands can be docked on a single GPU or 1,000 GPUs in about 8.3 hours, respectively.
Using Vina GPU 2.1 on Fovus, researchers can dock over 100x more ligands than CPU-based systems while maintaining cost efficiency. This approach accelerates high-throughput docking and enables docking of billions of ligands within reasonable timeframes. Leveraging spot instances and intelligent multi-cloud-region auto-scaling, Fovus ensures minimal cost even at large scales.
Optimizing Vina GPU 2.1 on Fovus
To demonstrate the optimality of Fovus, we ran tests using Vina GPU 2.1 on a protein-ligand docking task. The docking experiment used 1,000 ligands sourced from ChEMBL and ZINC20 databases and the Human Neurotensin hNTSR1 – Gi1 Protein Complex as the receptor. We tested AutoDock Vina GPU 2.1, QuickVina W GPU 2.1, and QuickVina2 GPU 2.1, running 30 tasks with 1,000 ligands each.
The Vina GPU software needs a config file specifying the required inputs, which is as follows:
receptor = /container_workspace/6os9.pdbqt
ligand_directory = /container_workspace/ligs
output_directory = /container_workspace/output
center_x = 127.854
center_y = 132.869
center_z = 124.8
size_x = 30
size_y = 30
size_z = 30
thread = 10000
Fovus uses the optimal HPC strategy AI determines, driven by benchmarking data, to ensure that each of the Vina GPU 2.1 methods runs at maximum efficiency. In high-throughput virtual screening, the large batch of docking simulations is independent and highly parallelizable, allowing for a significant reduction in time-to-insight through massive parallelization over hundreds or thousands of GPUs. As a result, minimizing the runtime of individual simulations is less critical, making cost efficiency the top priority. For this study, the docking job was submitted to Fovus with the objective of minimizing cost, prioritizing affordability over speed. Fovus evaluates all applicable HPC strategies on the AWS cloud and automatically selects the optimal one to minimize cloud costs while ensuring scalability and fast time-to-insight, delivering the most cost-effective solution without compromising the overall performance and computational efficiency.
Key performance metrics analyzed included:
- Total GPU-hours
- Average runtime per task (minutes) – which reflects the overall time-to-insight as all tasks ran in parallel across multiple GPUs.
- Total cost
30,000 Ligands Run Results
Vina GPU 2.1 Method | Total GPU-hours | Average Runtime per Task (minutes) | Total Cost |
---|---|---|---|
Autodock Vina GPU 2.1 | 81.2 | 162 | $30.11 |
QuickVina W GPU 2.1 | 26.5 | 53 | $9.88 |
QuickVina2 GPU 2.1 | 7.6 | 15 | $2.85 |
Our benchmarking results revealed the optimal runtime and cost that can be achieved across different methods:
- AutoDock Vina GPU 2.1: Resulted in an average runtime of 162 minutes per task and a total cost of $30.11.
- QuickVina W GPU 2.1: Achieved an average runtime of 53 minutes per task for a total cost of $9.88.
- QuickVina2 GPU 2.1: Demonstrated the fastest performance with an average runtime of 15 minutes per task and a total cost of just $2.85.
These results highlight the efficiency gains and cost advantages of using Vina GPU 2.1 on Fovus. QuickVina2 GPU 2.1 demonstrated the fastest performance and lowest cost, while QuickVina W GPU 2.1 provided a strong balance between cost and runtime for mid-scale docking projects. Overall, using Fovus enabled us to achieve significant speed increases and cost savings compared to traditional CPU-based approaches.
Unlocking Large-Scale Docking with Fovus
By leveraging Fovus to run Vina GPU, researchers can perform large-scale molecular docking tasks more efficiently and cost-effectively. With AI-driven optimization, dynamic scaling, and intelligent cost management, Fovus empowers scientists to tackle billion-ligand-scale projects without breaking the bank.
Get started with Vina GPU and experience the future of high-throughput molecular docking!